Researchers at MIT's Computer Science and Artificial Intelligence Laboratory (CSAIL) are turning science fiction into reality by developing a shape-shifting robot that resembles a shapeless slime, capable of performing various tasks without traditional mechanical components.
The MIT team's discovery involves a machine learning technique designed for a robot that doesn't have a conventional structure, such as arms, legs or skeletal supports, points out New Atlas.
Instead, this robot can change its shape by squashing, bending and stretching to interact with its environment.
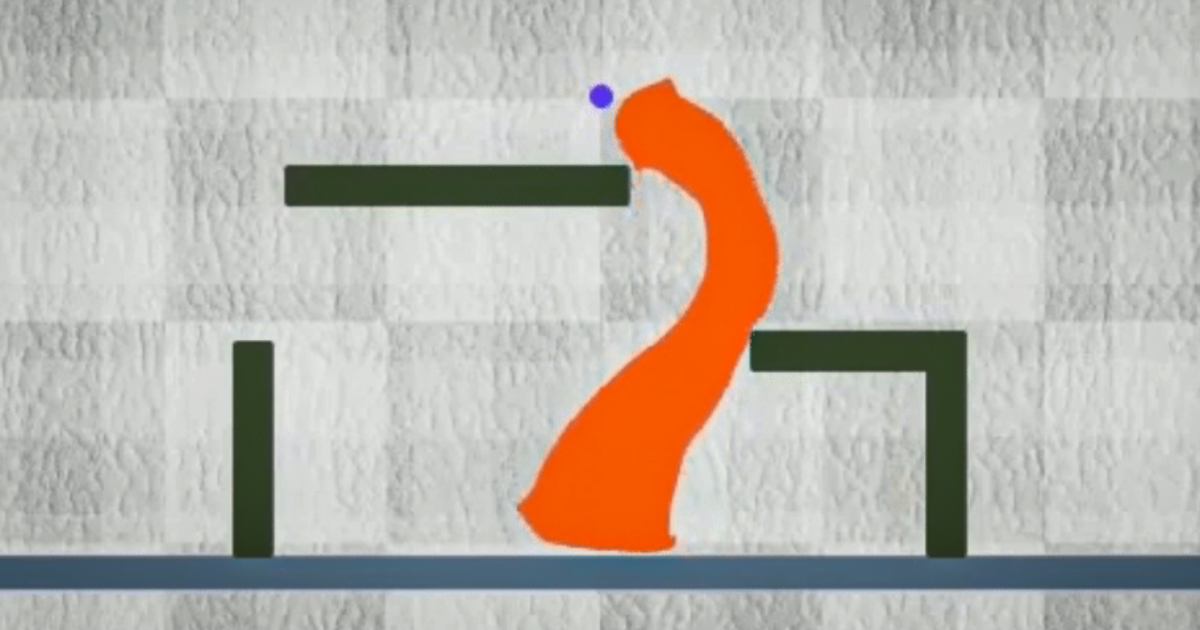
This innovation marks a significant departure from previous attempts at shape-shifting robots, which depended on external magnetic controls and were unable to move independently.
"When people think of soft robots, they tend to think of robots that are elastic but return to their original shape. Our robot is like slime and can actually change its morphology," points out Boyuan Chen, from MIT's Computer Science and Artificial Intelligence Laboratory (CSAIL) and co-author of the study available for consultation on arXiv.
"It's impressive that our method worked so well because we're dealing with something very new," adds Chen.
To manage this highly adaptable form, the team turned to artificial intelligence, taking advantage in particular of reinforcement learning to navigate the complexities of controlling such a versatile structure.
Reinforcement learning, normally used in more rigid and defined robotic systems, was uniquely adapted for this project. The researchers treated the robot's potential movements as an "action space", visualized as an image made up of pixels.
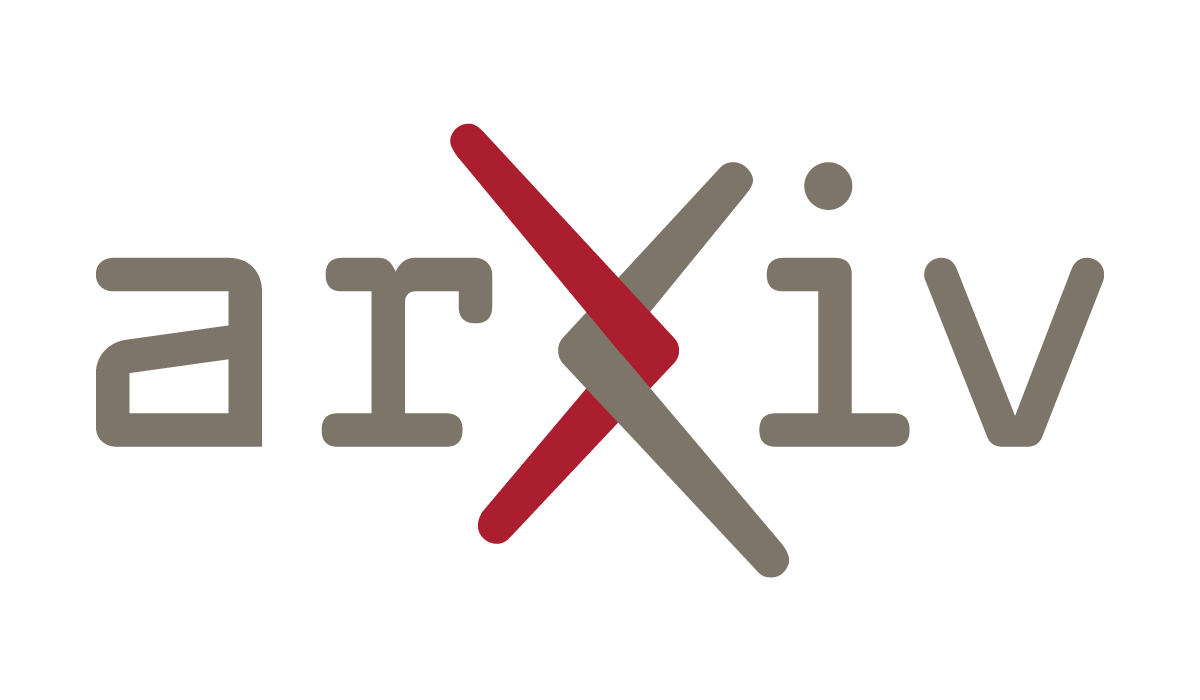
This method allows the robot to coordinate movements through what can be considered its "limbs", despite not having a fixed shape, allowing it to carry out coordinated actions such as stretching or compressing parts of itself.
To refine and improve the robot's movements, the researchers used a "coarse to fine policy learning" strategy. Initially, the algorithm works at a lower resolution, managing broader movements and identifying effective patterns.
It then moves to a higher resolution to fine-tune the actions, increasing the robot's precision and its ability to perform complex tasks.
The team tested their innovative control system in a simulated environment they developed, known as DittoGym.
This platform presented the robot with various challenges, such as shape matching or object manipulation, which are key to assessing the robot's adaptability and control efficiency.
Their findings showed that the coarse-to-fine approach significantly outperformed the other methods, providing a promising basis for further development.
Although practical real-world applications may still be some years away, the implications of this research are vast. Potential future uses of this technology could range from navigation in the human body for medical purposes to integration into wearable technologies.